The difficulty in fighting viral pandemics lies in the ability of these entities to evolve solutions to everything we throw at them. But what if we had a tool to predict what they were going to evolve before they did? By Tom Dubois.
On the 21st of September 1912, Harry Houdini was lowered head-first into a tank filled with water with his ankles locked into a mahogany lid. Upon entering the tank, the lid was locked onto the frame, and curtains were drawn to cover the contraption. As sombre music began to play, Houdini’s assistants stood on stage with axes ready to break the glass if necessary. Two minutes of suspense followed, when suddenly Houdini emerged from behind the curtains to thunderous applause.
Whilst Houdini’s displays of ingenuity and capacity for evasion were incredible, magical even, they are dwarfed in comparison to the tactics that viruses have evolved to evade their host’s immune system. Similar to how thieves might tamper with CCTV cameras before robbing a bank, many viruses can synthesise proteins that block the action of the complement system. This system is a non-specific host defence system used to tag pathogens for elimination and activate inflammatory responses (1). Some viruses such as HIV can cover themselves in a protective protein cloak called a capsid, which shields the virus from innate immune sensors capable of activating the interferon response (2) - an appropriate comparison for this evasion tactic can be thought of as Harry Potter’s Invisibility Cloak.
SARS-COV-2, the causative agent of COVID-19, is capable of modifying itself beyond recognition to evade our immune system. As an RNA virus, its surface proteins are capable of rapid evolution as the virus replicates and spreads through a population due to its extremely high mutation rates (3). Some of these, such as the Spike protein, are the targets of specific antibodies produced by our B lymphocytes in response to infection or vaccination. By binding to the Spike protein, antibodies neutralise SARS-CoV-2 by preventing entry of the virus into our cells. However, this creates a selection pressure for viruses which can evade recognition and binding of the antibodies.
Since SARS-CoV-2 mutates rapidly, mutants which are not recognised by the antibodies produced in response to a first infection evolve rapidly and have the potential for high propagation. This was one of the causes for the emergence of certain variants of concern (VOC) during the COVID-19 pandemic. The emergence of these variants was particularly concerning due to the discovery that certain vaccines were not as effective against some VOC (4). Consequently, a group of researchers at Harvard Medical School and Oxford set out to build an AI tool capable of predicting which mutations could enable SARS-CoV-2 to evade recognition by host antibodies.
The Oxford Applied and Theoretical Machine Learning Group (OATML) at Oxford, and the Debora Marks lab at Harvard had previously collaborated to create EVE, a model capable of predicting which protein variants from genes related to human diseases might be associated with pathogenicity (5). Upon realising that their work could be applicable to predicting immune escape by SARS-CoV-2, they repurposed their model to create EVEscape (6). This model uses a deep neural network trained on viral genomes derived from pre-pandemic coronavirus data sources, including sarbecoviruses such as SARS-CoV-1 and seasonal common cold viruses, as well as biophysical information to see how certain mutations can affect the structure and interactions of proteins. EVEscape predicts the probability that a viral mutation will induce immune escape by incorporating three terms. The first term calculates the probability that a mutation will not impact properties required for transmission (viral fitness), such as the protein’s expression, its ability to fold, and its capacity to bind to host receptors. The second term calculates the probability that the mutation occurs in a region accessible to antibodies. Finally, the third term calculates the probability that the mutation will disrupt antibody binding (fig. 1).
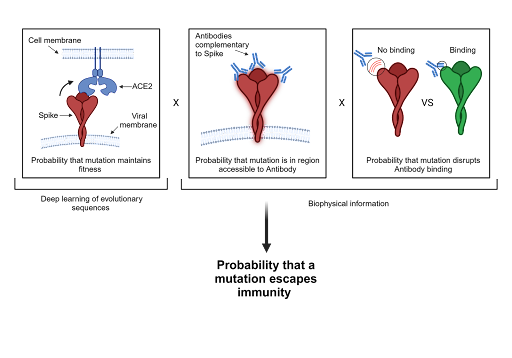
To test whether their model could really predict VOC before they appeared, the team ran a retrospective study on the COVID-19 pandemic - time-travelling to pre-January 2020 using information that was only available before the pandemic (spike sequences from Coronaviridae). This allowed them to test whether their model could predict which variants would be able to evade immune recognition and become VOC by focusing on the Spike protein’s Receptor Binding Domain, which has been shown to elicit a strong immune response. EVEscape was able to predict the appearance of significantly more VOC than previous computational methods. Furthermore, these attempts relied on pandemic sequences, antibody-bound spike structures, or both, which limited their predictive power early in pandemics compared to EVEscape. The model also fared well compared to experimental approaches used to determine immune evasion in emerging SARS-CoV-2 strains.
That being said, EVEscape is not without its limitations. Currently, when used independently, it cannot predict the appearance of all VOC. This may be due to the model not having sufficient knowledge of the exact constraints placed on a virus during a completely new pandemic. Therefore, EVEscape may best be used in conjunction with experimental approaches: for future pandemics, EVEscape could be used to flag probable VOC at early stages for experimental testing. This may be used to design vaccines less susceptible to immune evasion. As a pandemic progresses, EVEscape could be deployed to characterise newly emerging strains to design booster vaccines.
Something particularly cool about the model is that it is not limited to SARS-CoV-2. The team applied EVEscape to the surface proteins of the Lassa and Nipah virus, and found that it was also effective at predicting mutants known to cause immune evasion. This demonstrated the potential of the model for studying viruses under less surveillance than COVID-19, but which still have the potential to cause a pandemic. The model can also be generalised to influenza and HIV, and the escape mutants predicted by EVEscape have been released to aid vaccine development.
If you are interested in learning more about how EVEscape works and about its VOC predictions for SARS-CoV-2, here is the website where the EVEscape team releases biweekly VOC prediction reports: https://evescape.org/
And if you are interested to see the program of how the model works, here is the GitHub repository for the project: https://github.com/OATML-Markslab/EVEscape
Article written by Tom Dubois
References:
Cover photo by Miranda Hitchens, Technology and Engineering Editor
1. Alcami A, Koszinowski UH. Viral mechanisms of immune evasion. Immunology Today. 2000;21(9):447-55.
2. Zuliani-Alvarez L, Govasli ML, Rasaiyaah J, Monit C, Perry SO, Sumner RP, et al. Evasion of cGAS and TRIM5 defines pandemic HIV. Nature Microbiology. 2022;7(11):1762-76.
3. Steinhauer DA, Holland JJ. Rapid Evolution of RNA Viruses. Annual Review of Microbiology. 1987;41(1):409-31.
4. Souaid T, Hindy J-R, Kanj SS. What is Currently Known About the SARS-CoV2 Variants of Concern. Journal of Epidemiology and Global Health. 2021;11(3):257.
5. Frazer J, Notin P, Dias M, Gomez A, Min JK, Brock K, et al. Disease variant prediction with deep generative models of evolutionary data. Nature. 2021;599(7883):91-5.
6. Thadani NN, Gurev S, Notin P, Youssef N, Rollins NJ, Ritter D, et al. Learning from prepandemic data to forecast viral escape. Nature. 2023;622(7984):818-25.