Information overload? In the age of Big Data, researchers look to the nanoscale to handle it all. By Miranda Hitchens.
Perhaps the most conspicuous theme of our lifetimes have been the takeover of technology, as our economy undergoes a metamorphosis in the digital age. So-called ‘big data’ lies at the heart of these events, as the explosion of internet-enabled devices has incited ever-increasing streams of data, from your personal online footprint to constant inputs from sensors, smart assistants, texts, emails and images. However, is our technology equipped to process it all?
Up to this point, the progress of computing power was in part measured by the increase in transistor density, as predicted by Moore’s Law. While we have kept up with the forecast of the transistor population on a chip doubling approximately every two years, we have seen a stagnation in improvements to memory and speed. It is also intuitive that we can only keep up with Moore’s Law for so long, since transistors can’t get to subatomic scale. Traditional chip architecture also consists of separate processing and memory units, which creates the ‘von Neumann bottleneck’ - the principle that processing speed is limited by the time it takes to move data from memory to the processor.
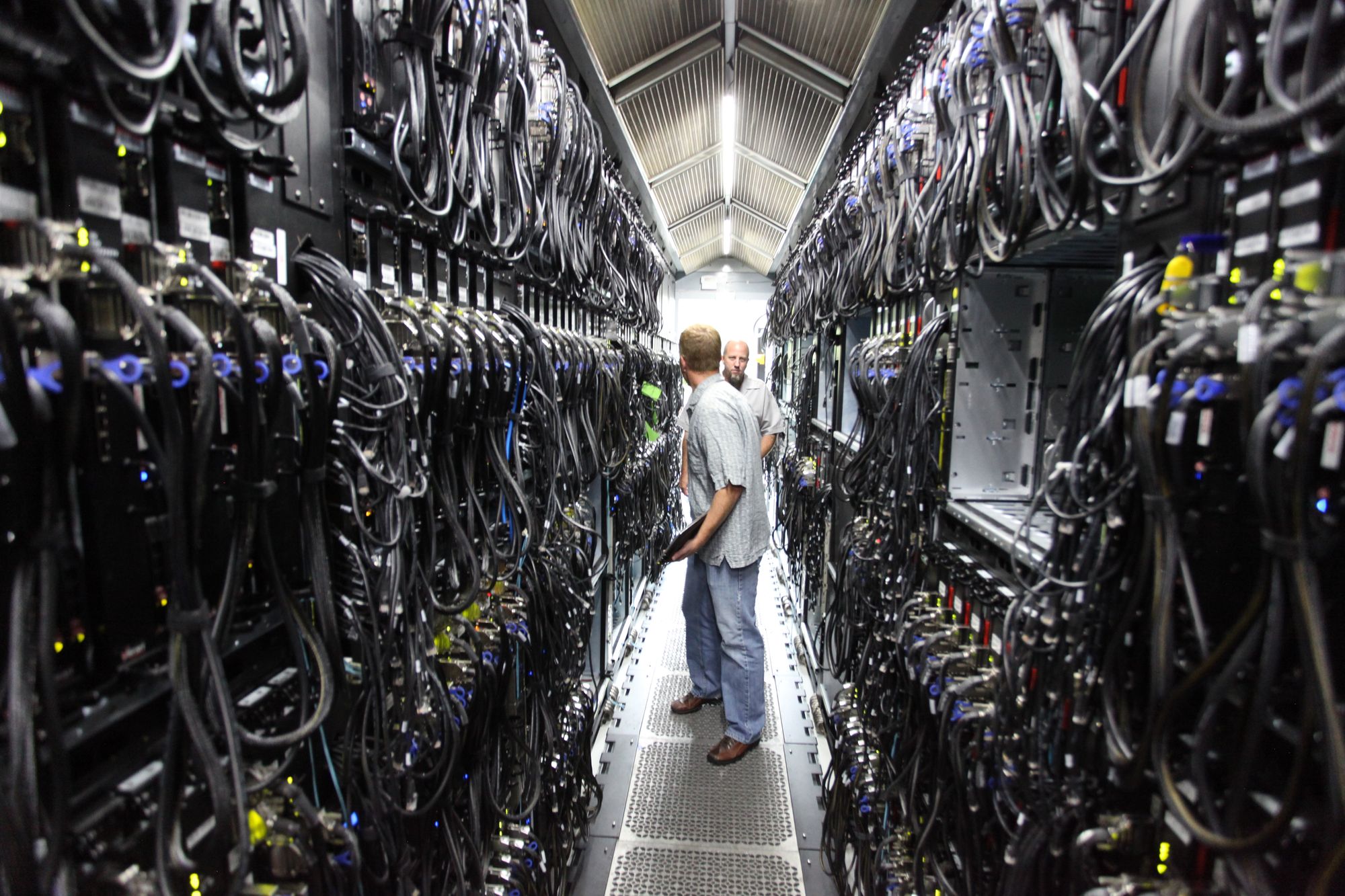
Meanwhile, researchers have identified that the amount of computational power required for our economic needs is rapidly increasing past the capabilities of traditional computer architecture, as well as imposing significant environmental costs due to the electricity required to operate large data centers. In order to sustain our rate of innovation in these areas, we may need to look beyond the computer architectures we have so long relied upon. Two of the most intriguing innovations in this field draw from the unexpected areas of biology and quantum mechanics, leveraging our increased understanding of physics to develop novel technologies.
One approach that has shown a lot of promise in accelerating processing at a much lower power cost is neuromorphic computing, which makes use of electrical analogs to emulate the structure and behavior of the brain. An integral component in achieving this is known as the memristor: a state-dependent device that exhibits similar behavior to a biological synapse [1]. The importance of state-dependence in this case is that the current state depends on the previous state, making its ‘weight’ effectively tunable. This is similar to how our brains work, as each synapse possesses a weight that is gradually tuned by the activity of electrical spiking, and is likewise state-dependent.
This mechanism has suited neuromorphic systems to run Spiking Neural Networks (SNNs), which use spikes to encode information, offering more degrees of freedom for computation: we can consider more factors than just the binary spike or no spike, such as the properties of spikes that vary with time, like phase and firing activity. However, these neural nets aren’t always applicable - standard Artificial Neural Networks can outperform SNNs in many areas of conventional machine learning. However, SNNs do offer an advantage in processing more challenging ‘noisy’ and time-dependent data [2], such as distinguishing smells and sounds. This also makes them interesting tools to potentially further our understanding of neuroscience by reverse engineering.
Another technology that strives to overcome the von Neumann bottleneck comes from the field of spintronics, which takes advantage of spin - the quantum mechanical angular momentum - of an electron, which produces a magnetic moment. The phenomena of Giant and Tunnel Magnetoresistance (GMR and TMR) use magnetic fields to induce resistance between ferromagnetic layers through separating electrons by their spin orientation.
A notable example of spintronic technology is MRAM, or Magnetic Random Access Memory. Almost anyone familiar with computers will know of RAM, which is the volatile memory that stores data currently being used by the computer, to reduce latency between the processor and the non-volatile memory. However, MRAM offers the possibility of non-volatile embedded memory, which can be read by measuring the resistance induced using TMR [3]. Additionally, the read and write speeds to MRAM are much greater than traditional memory, alongside lower idle power consumption and compatibility with logic devices we are already familiar with. This has great potential for use in small devices like smartphones, which depend on online connections to data centers for complex computation, causing a very high indirect power consumption [4].
While spintronics and neuromorphic systems are exciting prospects, we don’t yet have the ability to implement them and improve the efficiency of large scale data processing. However, the research is significant. Along with tech manufacturers rolling out MRAM devices and neuromorphic chips in recent years, we have an optimistic outlook for a future of low-power computing. Regardless of the ethics and our ability to fulfill its resource demands, Big Data is now a mainstay of our economy, and we may be better off taking a chance on new approaches than expensing ourselves and the environment to funnel endless power into data centers.
References
[1] Joksas, D. et al. “Memristive, Spintronic, and 2D-Materials-Based Devices to Improve and Complement Computer Hardware”, Adv Int Sys 4, 8 (2022) doi: 10.1002/aisy.202200068
[2] N. Imam, T. A. Cleland, “Rapid online learning and robust recall in a neuromorphic olfactory circuit”, Nat Mach Intell 2, 181–191 (2020). doi: 10.1038/s42256-020-0159-4
[3] Z. Guo et al., "Spintronics for Energy- Efficient Computing: An Overview and Outlook," Proceedings of the IEEE, 109, 8, 1398-1417 (2021), doi: 10.1109/JPROC.2021.3084997.
[4] Giovanni Finocchio et al, “The promise of spintronics for unconventional computing”, Journal of Magnetism and Magnetic Materials 521, 1 (2021), doi: 10.1016/j.jmmm.2020.167506.